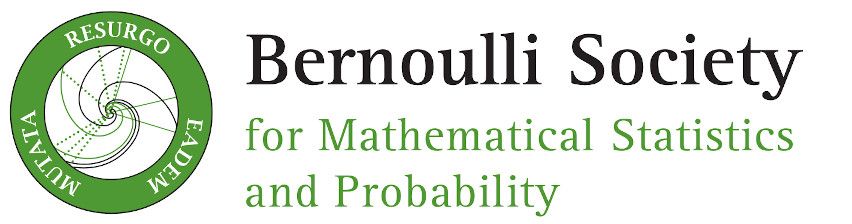
Workshop: Innovation in dynamic network modelling
We are pleased to invite you to join us for a workshop on “Innovation in dynamic network modelling”, held at Università della Svizzera Italiana on September 11-13, 2024. The workshop starts at 14:00 on Wednesday 11 September and finishes after lunch on Friday 13 September 2024.
This workshop centres around the latest advancements in dynamic network modelling, with a specific focus on innovation and patent citation analysis. It aims to cover a broad range of topics from relational event modelling to latent space modelling, providing a platform for discussing theoretical advancements and practical applications in dynamic network analysis.
Themes
The workshop will focus, broadly speaking, on Dynamic network modelling and, in particular, on three key themes:
- Relational Event Modeling
- Patent citations and innovation
- Latent space dynamic networks
The study of network structures has become increasingly important in fields such as economics, epidemiology, ecology, and biology. However, analyzing complex networks remains a challenge, even with big data.
Invited Speakers
(Confirmed so far)
Viviana Amati (Bicocca University)
Analysis of multiple independent sequences of relational event data
Traditionally, the analysis of relational event data tends to consist of case studies of one single sequence of relational events. In this work, we consider the multilevel analysis of relational events where the data consists of multiple sequences of relational events that are conceptually similar but are collected over disjoint sets of entities, and there are no events between them. The sequences can be regarded as independent replications of the same event dynamics concerning the social processes that have generated the events. This type of data derives, e.g., from the collection of relational event sequences over distinct sets of units belonging to different geographical areas or replications of studies on various groups of entities. We develop procedures to jointly analyze multiple sequences of relational events that are independent and to determine the extent to which the event dynamics generalize to different contexts and across various groups. In particular, we discuss several approaches, including meta-analysis and the use of random coefficient models, and investigate in which situations one method is more suitable using simulations. We illustrate how to apply the proposed procedures by using sequences of relational events describing collaborations between academics in different disciplines.
Laurence Brandenberger (ETH Zurich)
Advancements in Relational Event Models: Unveiling Network Dynamics Through Interaction Effects, Temporal Analyses, and Causal Insights
Relational Event Models (REMs) provide a robust framework for analyzing the evolution of network effects over time by leveraging event sequences — network events ordered chronologically — to elucidate intricate dependencies and evolving patterns. This presentation outlines three pivotal advancements in relational event modeling.
First, we introduce the concept of interaction effects, offering nuanced insights into network dynamics by revealing localized phenomena within the network structure. For example, our analysis demonstrates a heightened tendency towards reciprocity within right-wing parties in parliamentary settings, juxtaposed with a tendency for left-wing parties to refrain from reciprocating favors.
Second, we demonstrate the capacity of REMs to track temporal changes in network dynamics. Employing a sliding time-window approach, we can observe how network effects evolve over time and adapt in response to external stimuli or events.
Third, we showcase the potential of REMs to uncover causal relationships through temporal permutations applied to event sequences. By disrupting the temporal order of events, we disentangle genuine dependencies — such as social influence — from static behavioral traits, such as homophily or selection effects.
These three innovations afford insights into network dynamics, opening up new avenues for the investigation of social patterns.
Jürgen Lerner (University of Konstanz)
Relational hyperevent models for citation networks
Scientific networks can represent the co-evolution of coauthor networks, connecting scientists to the papers they publish, and citation networks, connecting papers to their references. A single published paper gives rise to a hyperedge, simultaneously connecting varying and potentially large numbers of authors and references. In this talk I discuss relational hyperevent models (RHEM) for the joint modeling of collaboration and citation networks. RHEM can account for polyadic dependencies in the data, i.e., interactions among more than just two nodes, which cannot be well represented by a purely dyadic specification of the event rate. An analysis of empirical networks comprising millions of authors and papers reveals that such hyperedge covariates yield some of the strongest effects explaining the reference lists of published papers. I briefly overview practical aspects, including computational issues, in specifying and estimating RHEM with the open-source software eventnet (https://github.com/juergenlerner/eventnet).
Catherine Matias (Sorbonne University)
A semiparametric extension of the stochastic block model for longitudinal networks
We propose an extension of the stochastic block model for recurrent interaction events in continuous time, where every individual belongs to a latent group and conditional interactions between two individuals follow an inhomogeneous Poisson process with intensity driven by the individuals’ latent groups. We show that the model is identifiable and estimate it with a semiparametric variational expectation-maximization algorithm. We develop two versions of the method, one using a nonparametric histogram approach with an adaptive choice of the partition size, and the other using kernel intensity estimators. We select the number of latent groups by an integrated classification likelihood criterion. We demonstrate the performance of our procedure on synthetic experiments, analyse two datasets to illustrate the utility of our approach, and comment on competing methods.
Veronica Vinciotti (University of Trento)
Causal relational event models
Causality plays an important role also in network science, where many questions can be phrased in terms of detection of the causal drivers of network formation. Despite this, causal inference has not received much attention in this field. In this talk, I will discuss causality in the context of relational event models for time-stamped interaction data. Whereas maximum likelihood estimation of such models provides the best in-sample risk, with limited out-of-sample guarantees, a causal relational event model would have the best out-of-sample guarantees. As the nested case-control partial likelihood of a relational event model is a special case of a generalized linear model, we show that the causal parameter can be written as a conditional maximum likelihood estimator subject to a Pearson risk constraint that imposes causal stability.
Ernst Wit (Università della Svizzera italiana)
What is next in modelling dynamic networks?
Stochastic Actor Oriented Models, STERGMs, DyNAMs and REMs have opened up the field of dynamic network modelling in a way that is often much more adequate for applications than static network models. Technical, computational and conceptual advances in the past 15 years have allowed the methods to be applied to an ever wider range of applications, not constrained by sample size or model complexity. In this talk I will touch upon a number of developments for particularly the relational event model and dynamic network actor models.
From a computational point of view, DyNAMs and REMs can use standard Poisson regression implementations to evaluate the full likelihood, as long as events are dense, and the assumption of piecewise constant hazards is satisfied asymptotically. Alternatively, the logistic regression implementation of the nested case control version of the partial likelihood, can be improved arbitrarily by sampling multiple non-events, while keeping the convenience of the logistic computational framework.
From a conceptual point of view, one of the main upcoming challenges will be dealing with multiplex and hyper event networks. These types of networks are becoming increasingly relevant but have only recently been considered from a dynamical perspective.
From a data perspective, network sampling not always records the instance of an event. Often, events are clustered in larger units, violating the single event assumption, or cross-sectional samples at various time points are available. Although certain models (such as the SOAM) have considered these types of data before, this has not been done for REMs and DyNAMs.
Venue
The workshop will be held at the Institute of Computing, Faculty of Informatics, Università della Svizzera Italiana. Via la Santa 1, Lugano.
Abstract Submission and Registration
Our workshop invites submissions for poster or contributed talks to be held in-person.
Please submit your abstracts using the link below and register by May 15th May 31st. Although there is no fee for registration, which includes meals for on-site attendees, we ask you to indicate your planned attendance days for adequate preparations. Acceptance confirmation emails will be sent starting May 16th.
To register for the workshop, please follow this registration link. For those unable to join us physically, we are offering the option to attend the sessions online. Online attendees will receive access details at the email used in the registration closer to date.
We look forward to your participation in this exciting workshop!
Accommodation info
You can secure your accommodation by booking a room at Hotel Ceresio (Via Serafino Balestra 19, 6900 Lugano, Svizzera). Until the end of May, you can take advantage of discounted prices for single (90 CHF) or double rooms (120 CHF, subject to availability) by contacting them via email at info@hotelceresio.ch. You can simply mention “USI conference booking 11-13 September” (or “Prenotazione conferenza USI 11-13 Settembre”) in the subject of your email.
Program
Schedule of the workshop can be found here:
Contributed Talks
Session 1 – Effects in Relational Event Modeling
Rūta Juozaitienė (Vytautas Magnus University)
It’s about time: revisiting reciprocity and triadicity
Relational event models are event history models for dynamic network interactions. Computational considerations have resulted in frequently used simplified network statistics. This study explores the impact of diverse endogenous effect definitions. Simulations and real-world studies focused on reciprocity and transitivity effects emphasize the need for more complex effect definitions to avoid possible contradictory interpretation of the results. We introduce a flexible computational framework to infer such effects efficiently.
Alvaro Uzaheta (ETH Zurich)
Modeling heterogeneity in social media data using DyNAMs
The increasing availability of fine-grained social interaction data from digital sources presents new challenges for researchers using relational event models. This data is often characterized by different sources of variation, including time and actor heterogeneity, that are averaged out when relational event models with fixed coefficients are applied.
In this work, we present an extension of the Dynamic Network Actor Models (DyNAMs) that accounts for the variation of coefficients over time and across actors through time-varying coefficients and random effects, respectively. Time-varying coefficients are modeled using a Hidden Markov chain with a predefined number of regimes, capturing variations due to non-observable, time-varying variables or latent patterns. Random effects across actors account for unobserved heterogeneity in actors’ preferences and activities, attributable to unobserved attributes or unspecified network statistics.
We demonstrate the applicability of this framework by analyzing the “liking” relations among aspiring and professional designers on a social media site. The model’s flexibility facilitates the study of user behavior and its evolution over time, considering deviations from the average behavior.
Melania Lembo (Università della Svizzera italiana)
Relational event models with global covariates
Traditional inferential techniques for Relational event models involve Cox’s partial likelihood and can estimate the effects of covariates that are node-specific, such as age or in-degree, or dyadic, such age difference of pairs of nodes or reciprocity. However, they cannot account for global covariates, i.e., variables that are constant for all pairs (e.g. weather or time of the day). Indeed, these covariates, being only time-dependent, drop out from the partial likelihood. Nevertheless, they are often important in capturing and explaining the temporal nature of the relational events under study. In this talk, such a challenge is addressed with the use of nested case-control sampling on a time-shifted version of the event process. This will result in a likelihood of a degenerate logistic generalized additive model from which we are able to recover effects of all kinds of covariates, including global ones.
Session 2 – Innovation
Anuška Ferligoj (University of Ljubljana)
Analysis of the temporal sequence of co-authorship networks between European countries in the years 1990-2023 from OpenAlex.
There were many research initiatives in the European Union to speed up the scientific collaboration between EU countries. By analyzing the temporal sequence of co-authorship networks between European countries, we will analyze the effects of those initiatives.
The data were obtained using OpenAlex (https://docs.openalex.org/) – a fully open catalog of the global research system. It is named after the ancient Library of Alexandria and it is made by the non-profit OurResearch (https://en.wikipedia.org/wiki/OurResearch). Batagelj developed a package of functions OpenAlex2Pajek in R (https://github.com/bavla/OpenAlex) for constructing bibliographic networks from selected bibliographic data in OpenAlex. Using OpenAlex2Pajek the temporal sequence of co-authorship networks between EU countries in the years 1990-2023 was constructed. Several approaches to blockmodeling these temporal co-authorship networks will be compared and discussed. This talk is based on joint work with Vladimir Batagelj, Marjan Cugmas and Franc Mali.
Vladimir Batagelj (IMFM Ljubljana and IAM UP Koper)
Longitudinal approach to the analysis of temporal networks based on temporal quantities
Early applications of temporal networks were introduced in project scheduling (CPM, Pert) in operations research, in transportation network analysis, and constraint networks in artificial intelligence. Also, for data analytic tasks, different approaches were proposed (Holme and Saramäki, 2023). Most frequently the cross-sectional approach is used in which the time consists of a finite number of time points (intervals). A time slice for a given time point t is a (static, ordinary) network for which the set of nodes/links contains all the nodes/links active in this time point. The analysis is usually performed on each slice separately using standard network analysis methods producing a ‘time series’ of results. Another interesting formalization is the time-varying graphs (Casteigts, Flocchini, and Quattrociocchi, 2012).
In the paper, Batagelj and Praprotnik (2016) proposed a longitudinal approach to the analysis of temporal networks based on temporal quantities. It is an alternative to the traditional cross-sectional approach. A temporal quantity (TQ) describes how the corresponding property changes through time. The proposed approach has the following advantages: (1) it works for both discrete and continuous time (2) it internally (inside operations) adapts to the granularity of data (3) the result of a method is usually again a temporal network or a list of temporal quantities.
We will present a formal framework for temporal analysis of networks based on TQs. In the example of bibliographic data, we present two ways (instantaneous and cumulative) to create the corresponding temporal bibliographic networks based on TQs. For illustration, some examples of their analysis will be given. The proposed approach is supported by the Python library TQ (https://github.com/bavla/TQ).
Edoardo Filippi-Mazzola (Università della Svizzera italiana)
Discovering Innovation Patterns in the US Patent Citation Network through the Deep Relational Event Additive Model
The dynamic network formed by patents citing each other offers valuable insights into how this relational system evolves and how innovation patterns change over time. However, modeling these networks presents significant challenges due to their large scale, making efficient modeling a computationally intensive task. This challenge is further compounded when incorporating non-linear effects for various covariates. To address these issues, we introduce the Deep Relational Event Additive Model (DREAM), which allows each non-linear effect in Relational Event Models to be captured by an independent neural network. By strategically balancing computational complexity with improved memory management and leveraging the capabilities of Graphics Processing Units (GPUs), DREAM efficiently captures complex non-linear relationships within the data. Applied to U.S. patent citation data from 1976 to 2022, encompassing over 8 million patents and 100 million citations, DREAM revealed key citation patterns, providing crucial insights into current innovation dynamics.
Federica Bianchi (Università della Svizzera italiana)
Network Effects and Evolving Knowledge Landscapes
This presentation explores the interplay between networks and innovation processes. I examine how endogenous and time-dependent network effects shape innovation trajectories. I focus on the evolving nature of knowledge creation and diffusion, analyzing innovation-related phenomena like shifts in knowledge composition and the declining similarity among patented inventions. Finally, I will also explain how we extended the REM framework to better capture the inherent temporal dynamics of these time-dependent network effects, thus enabling a deeper understanding of the complex and dynamic nature of decentralized systems.
Session 3 – Dynamic Network Modeling and Hypernetwork Embedding
Sonja Petrović (Illinois Institute of Technology)
Longitudinal Network Models, Log-Linear Multigraph Models, and Implications to Estimation and Testing Model Fit
We study longitudinal networks whose edges turn on and off according to a discrete-time Markov chain with exponential-family transition probabilities. We characterize when their joint distributions are also exponential families with the same parameters, and show that the permutation-uniform subclass of these chains permit interpretation as an independent, identically distributed sequence on the same state space. We apply these ideas to temporal exponential random graph models, for which permutation uniformity is well suited, and discuss mean-parameter convergence, dyadic independence, and exchangeability.
The framework facilitates applying standard tools to longitudinal-network Markov chains from either asymptotics or single-observation exponential random graph models.
The latter are often in log-linear form, allowing us to frame the problem of testing model fit through an exact conditional test whose p-value can be approximated efficiently in small and large networks. An important extension of this theory is to latent-variable block models, an application which will be briefly discussed.
This talk is based on joint work with William K. Schwartz, Hemanshu Kaul, Despina Stasi, Elizabeth Gross, Debdeep Pati, and Vishesh Karwa.
Fabio Ashtar Telarico (University of Ljubljana)
Comparison of Stochastic Block models for Dynamic Networks: An Empirical Study of the Global Trade Network
Problem statement: Datasets containing network data with a prominent temporal dimension are allowing researchers to provide novel models of networks’ evolution over time. It is especially true in relation to a network-clustering techniques called stochastic blockmodeling (SBM). However, drawing inference on the pattern of ties or temporal (or ‘dynamic’) networks using SBM can be challenging in empirical applications. Not least because of the large array of sound methods and differing conceptualisations the literature provides and of the fact that available methods have been employed mostly on binary networks. Whereas many of the networks in the social and economic sciences are valued/weighted by nature – chiefly, in the context of this presentation, the global trade network (GTN). Furthermore, the conceptualisation of network underlying each of the available SBMs reveals the sort of relations they were thought to model: food webs, interpersonal relations of various kind, organisational matters, and so on. Many of these networks exhibit features such as transitivity, the Matthew effect, and (approximate) equality of relational capability across the units. Whereas the GTN follows its own governing logic and mechanisms for change that depend, to a great extent, on theoretical assumptions. Moreover, edges’ incidence and their value may follow different processes. Thus, it is unclear whether existing SBMs can effectively model the GTN’s evolution over time.
Objective: This presentation reports on the comparison between existing implementations of SBMs for dynamic networks. Although the literature already provides a comparison of this sort, this is the first systematic attempt at comparing the performance of several SBMs on empirical data that does not fit with the underlying conceptualisation of network and lies beyond the method’s original use-case .
Methods: The methods are compared based on several versions of the GTN. In particular, selected SBMs for dynamic networks are applied on data from the UNCTAD database are used to describe the evolution of trade according to the tenants of two competing views of international trade. One the one hand, New Trade Theory, which predicts trade concentrates between countries that are similar in terms of productivity and factor endowments. In this view, trade flows should be considered as a high-level aggregate to better capture the effects of increasing returns to scale in monopoly-prone market niches. On the other, World-System Analysis and Dependency Theory, which argue that international trade is an intrinsically unequal exchange that deprives poorer parts of world of their (mostly natural) wealth while imposing the import of expensive high-valued-added products manufactured in wealthier countries. Hence, trade should be disaggregated to distinguish the extraction outflow of commodities from the high-value-added outflow of manufactured products.
Expected results: The choice of trade networks as the study’s focus relies on the acknowledgment that this type of relational data exhibits higher order dependency (e.g., reciprocity) and, thus, clusterability. However, clustering techniques bring with them different and sometimes diverging assumptions. Thus, the expectation is that SBMs may cluster units based on local tie-generating mechanisms (e.g., gravitational effects) in the aggregated trade network. Whereas, by distinguishing between commodities, the methods should more easily identify global mechanisms that underly the centre-periphery structure of unequal exchange. However, the influence of different tie-weighting methods (incl. binarization) is quite unpredictable albeit they may play a key role in normalising countries’ differing relational capacities.
Aleš Žiberna (University of Ljubljana)
A Markovian approach to stochastic blockmodeling of dynamic “snapshot” networks
A new stochastic blockmodeling approach for dynamic “snapshot” networks is presented. The aim of the approach is to be especially useful for cases where the blockmodel changes in time, as previous work has shown that in such cases existing method for blockmodeling of dynamic snapshot networks are often surpassed by more general blockmodeling approaches (e.g. those for blockmodeling linked/multipartite networks).
Our approach takes into account which units are the same in different time points and assumes that the dynamics between clusters is governed by some probabilistic Markov-type laws. For each unit, we therefore assume that its membership in certain clusters at different time points is influenced by the cluster membership at the previous time point, but not by the clusters before that. Within each time point, the links between units follow the probabilistic laws arising from cluster membership in a similar way to the previously proposed models. The additional information in the form of connections between time points aims to reveal a more meaningful and stable cluster structure. Our model does not assume that the clusters are the same at different time points or that their number is the same. In addition to the standard clusters, we introduce two special virtual clusters, which we call “emerging” and “vanishing” clusters. Their task is to model the distribution over the clusters for those units that appear at a later time or vanish at a certain time. The transitions from these artificial clusters amount to modelling the a priori cluster membership of new units and the exit rates by cluster, which adds flexibility to our model.
Kalle Alaluusua (Aalto University)
Hypergraph embedding for consistent line clustering
We examine the evolving topic of hypergraph embeddings by addressing the problem of line clustering. Line clustering considers grouping sets of points in a Euclidean space so that each group aligns well with a single line segment. We transform the line clustering problem into a problem of recovering communities within hypergraphs. Specifically, for every set of three points, we compute a line of best fit and treat triples whose deviation from this line is below a threshold parameter as hyperedges. This embeds the underlying line structure into a hypergraph. We derive information-theoretic bounds that characterise the setting where the exact recovery of the underlying clusters succeeds under a generative model with Gaussian noise. We further investigate hypergraph clustering algorithms to group the points from the constructed hypergraph. Unlike traditional hypergraph block models, the hyperedges so constructed are not independent, which complicates the theoretical analysis of the algorithm. This project not only motivates hypergraphs that incorporate geometry but also examines a framework to address challenges arising from dependent hyperedges in other statistical learning applications.
Session 4 – Advancements in Relational Event Models
Rumana Lakdawala (Tilburg University)
Duration Matters: A Relational Event Approach for Jointly Modeling Event Rates and Event Duration
The study of relational events, which are interactions occurring between actors over time, has gained significant traction in various fields such as social network analysis, organizational behaviour, and communication studies. Traditional relational event models typically focus on modelling the occurrence and sequencing of events without considering their duration. We introduce a novel Duration Relational Event Model (DREM) that incorporates the temporal duration of events into the analysis. The proposed model extends the existing framework by i) allowing one to accounting for how the duration of past events affects future interactions rate and ii) how the past event history and covariates affect the duration of future events.
Maria Eugenia Gil Pallares (Social Networks Lab, ETH Zurich)
Expectation Maximization for the estimation of parameters in relational event models with missing sequence information
Relational event models (REMs) are statistical models for the analysis of sequences of relational observations in social networks. Initially, they were applied to study, for example, social mechanisms in phone call records or interactions on social media platforms. These mechanisms may refer to exogenous covariates (e.g., the similarity between individuals), endogenous effects (e.g., patterns of reciprocation and clustering), and temporal aspects (e.g., for how long incoming interactions influence future interactions). The estimation of such models is straightforward (but potentially computationally challenging) when the exact sequence of events is known, as in the case of phone call records. However, sequences of relational event data may be partly missing or difficult to collect in the first place. This may be the case when online platforms only provide aggregated data on user behavior or when networks are considered that do not represent behavioral data but cognitive perceptions of relationships, as in the case of friendship perceptions.
We present an application of the Expectation Maximization algorithm to the estimation of parameters of one REM variant: the Dynamic Network Actor Model (DyNAM) with an unknown sequence of events. The Expectation step is computationally intractable and approximated by random samples of plausible event sequences. Preliminary results reveal that the EM-DyNAM is sensitive to the initial sample of sequences used during the estimation. The extension of the algorithm thus will be rooted in the Stochastic Approximation Expectation Maximization literature, where EM is combined with Evolutionary Monte Carlo, to help explore the sampling space and avoid the dependency of the initial conditions.
The talk introduces a statistical procedure for the estimation of parameters in case of missing information on sequences, discusses strategies for a computationally efficient implementation, and presents an applied case study. The model partly replicates the stochastic actor-oriented model (SAOM) for longitudinal panel data. However, some model features are distinct and systematically compared to results of a SAOM model on the same data. Those features include the introduction of Evolutionary Monte Carlo to accelerate the sampling phase, different specifications of the rates, the possibility to include continuously measured exogenous variables, and a different approach to separating tie creation and deletion processes.
Martina Boschi (Università della Svizzera italiana)
Goodness of fit of additive mixed-effect relational event models
Dynamic networks involve interactions that are temporally ordered, with past configurations influencing future ones. Relational event models help reveal these dynamics, but assessing their fit – especially when time-varying or random effects are involved – can be complex and computationally intensive.
We propose an additive mixed-effect relational event model estimated via case-control sampling, and introduce a versatile framework for testing its goodness of fit via weighted martingale residuals. Our technique relies on a Kolmogorov-Smirnov type test to evaluate covariate modeling and can be adapted for other network dynamics. We validate our approach using synthetic data and we apply it to analyze 57,791 emails among employees at a Polish company. Implementation is performed using R package mgcv.
Posters
Veronica Poda (University of Trento)
Comparison of modularity-based approaches for nodes clustering in hypergraphs
Ester Riccardi (University of Trento)
Unravelling the mystery of causal relational event models
Lovnesh Bhardwaj (Università della Svizzera italiana)
Factors Influencing the Citation of a Patent in Another Patent Modelled through REMs
Organising Committee
Prof. Dr. Ernst Wit, Dr. Francisco Richter, Dr. Federica Bianchi, Martina Boschi, Edoardo Filippi-Mazzola, Melania Lembo.
For inquiries or further information, please contact Melania Lembo at melania.lembo@usi.ch.
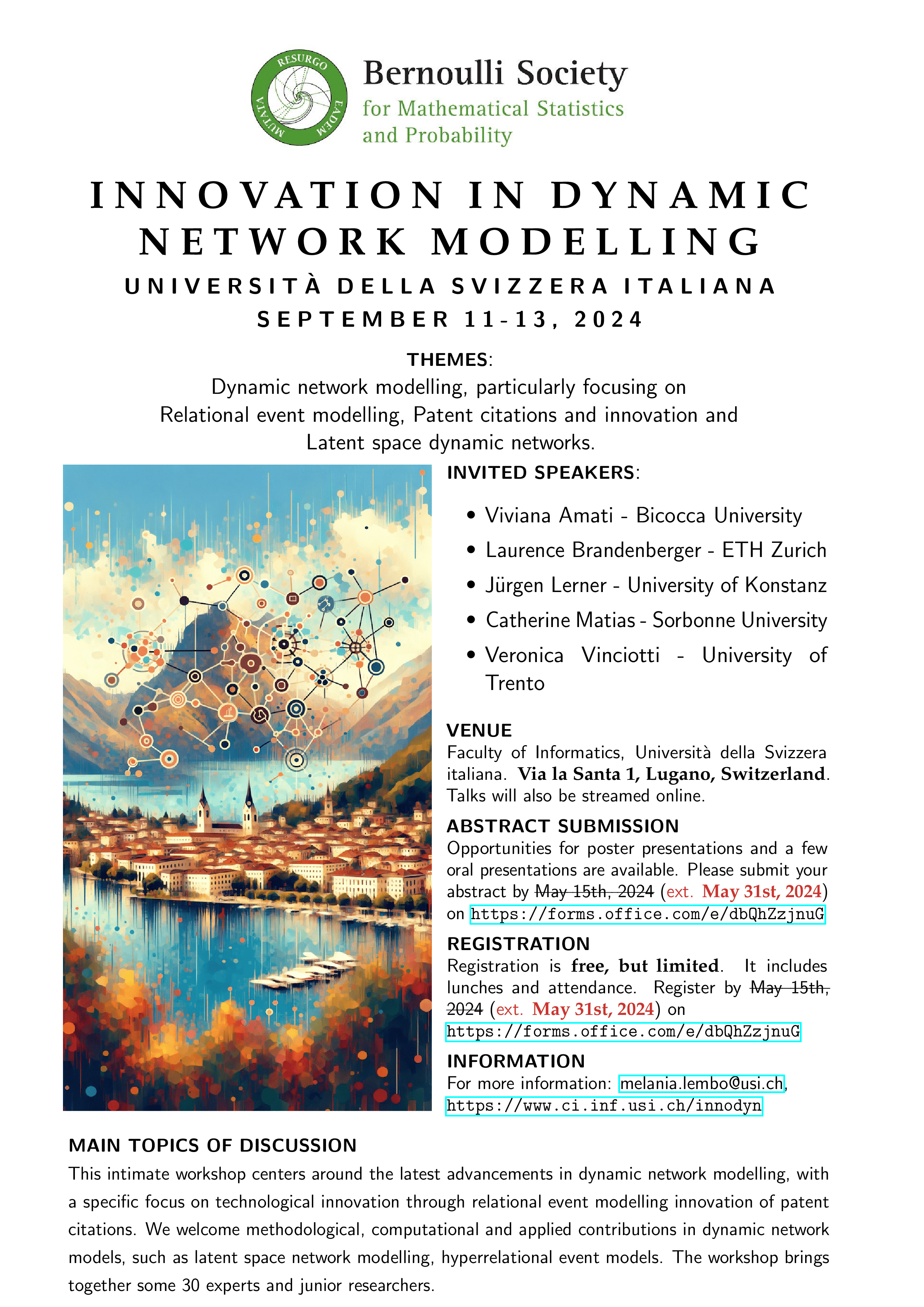